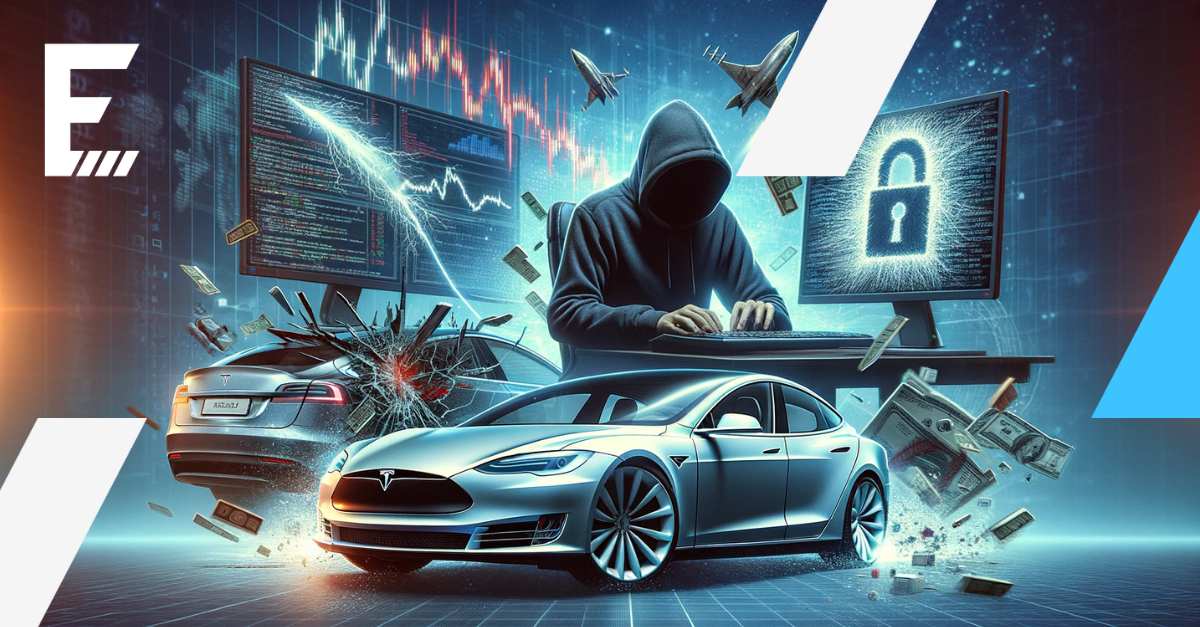
In the evolving realm of cybersecurity, insider threats represent a particularly insidious risk. Unlike external attacks, these threats originate from within an organization, often from individuals entrusted with access to sensitive systems and data. The recent data breach at Tesla, as reported by Gizmodo, offers a poignant case study of such risks. This article will delve into the various facets of insider threats, emphasizing the importance of advanced network monitoring tools like Netflow in detecting and mitigating these risks.
The Perilous Nature of Insider Threats
Cybersecurity risks are diverse and multifaceted, but insider threats stand apart due to their origin. These threats are not the work of external hackers probing for vulnerabilities from afar. Instead, they arise from individuals within an organization—employees, contractors, or business partners—who have legitimate access to the organization's network and data. This access, coupled with the insider's knowledge of the company's security practices and business operations, renders these threats challenging to detect and potentially devastating in their impact.
Access and Familiarity: Insiders, by virtue of their position, have direct access to critical systems and data. This privilege allows them not only to bypass many of the external defenses the organization has in place but also to understand and exploit weaknesses in internal controls. For example, an employee in the finance department might have unrestricted access to sensitive financial data and systems, which they could misuse for personal gain.
Detection Challenges: Detecting insider threats is a complex task. The activities of a malicious insider often mimic legitimate operations, making it difficult for traditional security tools and protocols to flag them as suspicious. For instance, a system administrator downloading large volumes of data might be performing a routine backup, or they could be stealing confidential information. Distinguishing between these scenarios requires more than basic security measures.
Potential for Greater Damage: Insiders, aware of the most valuable data and critical systems within an organization, can target their attacks more precisely than external hackers. This targeted approach can lead to more significant damage, as the attacker can focus on extracting or compromising the most sensitive information, leading to substantial financial losses, reputational damage, or even jeopardizing the safety of employees and customers.
Vulnerabilities to Insider Actions
Despite having robust external defenses, many organizations find themselves particularly vulnerable to insider threats due to several factors:
Privileged Access: In most organizations, certain employees have elevated access rights to perform their duties effectively. IT staff, executives, and others in sensitive roles often possess such privileges, potentially offering a broader surface for internal attacks. For example, an IT technician with access to network configurations could alter settings to create vulnerabilities or to cover their tracks.
Lack of Internal Monitoring: A common shortfall in many organizations is the lack of a comprehensive internal monitoring system. While external threats are often well-guarded against, the internal network activity might not be scrutinized to the same extent. This oversight can leave a blind spot for insider threats, where malicious actions go unnoticed until it's too late.
Inadequate Training and Awareness: Another factor contributing to organizations' vulnerability to insider threats is the lack of adequate training and awareness among employees. Many internal breaches occur not from malice but from ignorance or negligence. Employees might fall prey to phishing attacks, share passwords, or leave their devices unsecured, inadvertently becoming a conduit for insider threats.
Unraveling the Complex Web of Insider Activity with NetFlow
NetFlow has become an integral tool in network monitoring and threat detection. This protocol collects data about the traffic flowing through a network, providing valuable insights that can be used to detect unusual patterns or anomalies indicative of insider threats.
NetFlow facilitates the analysis of network traffic by logging data packets' metadata as they traverse the network. This data includes source and destination IP addresses, port numbers, the protocol used, and other pertinent details. By analyzing this data, NetFlow enables the identification of standard traffic patterns within an organization and, more importantly, the detection of deviations from these patterns. For example, if an employee usually accesses certain servers during specific times of the day, any significant deviation from this pattern could signal an anomaly worth investigating.
While many NetFlow collection solutions require sampling, meaning only a subset of traffic data is captured and analyzed, raw unsampled NetFlow goes a step further. It captures comprehensive data on every packet that moves through the network, offering a complete view of network activity. This level of detail is crucial in detecting the subtle and sophisticated maneuvers of insider threats. For example, an insider might slowly exfiltrate data over an extended period to avoid detection. Analysis of raw unsampled NetFlow can pick up on these small but consistent anomalies.
Tesla's Data Breach: A Case Study in Missed Opportunities
The recent data breach at Tesla offers valuable lessons in the importance of comprehensive network monitoring. Tesla's choice of a network monitoring solution reportedly does not recommend raw unsampled NetFlow due to scalability constraints. This limitation likely impeded Tesla's ability to fully monitor and analyze their network traffic, potentially delaying the detection of abnormal data movements indicative of an insider breach.
The absence of raw unsampled NetFlow in Tesla's network traffic monitoring likely meant that only a fraction of the network activity was under scrutiny. Consequently, subtle signs of the breach might have gone unnoticed, allowing the perpetrator more time to access and potentially exfiltrate sensitive data. For instance, if an insider was slowly gathering data over several weeks, the lack of detailed network traffic analysis might have allowed this activity to blend in with normal operations.
ElastiFlow: A Superior Alternative for Insider Threat Detection
Update June 2024: Since publishing this post, ElastiFlow has launched NetIntel, a product that enhances and enriches flow data with significantly more threat intelligence information.
ElastiFlow gives users the ability to collect and analyze unsampled NetFlow. As a result, it’s an extremely powerful solution for insider threat detection. By combining raw unsampled Netflow with ElastiFlow’s machine learning-based anomaly detection, users can significantly enhance their organization's ability to detect insider activities that may be nefarious or malicious.
Comprehensive NetFlow Visibility: ElastiFlow's support for raw unsampled Netflow ensures that every data packet is analyzed, leaving no gap in monitoring. This comprehensive analysis is key to detecting insider threats, as even the smallest anomaly could indicate a breach. By scrutinizing every aspect of network traffic, ElastiFlow provides an unmatched level of visibility into potential insider activities.
Managing High Data Volumes with Efficiency: One of the challenges of handling raw unsampled NetFlow is the vast amount of data generated, which can be overwhelming for many systems. ElastiFlow leverages Elasticsearch (and other Open Data Platforms) as its datastore to handle large volumes of network data efficiently. Additionally, ElastiFlow utilizes Elasticsearch's Time Series Data Stream (TSDS) capabilities, which optimizes data storage and retrieval, reducing the required storage capacity by up to 60%. This efficiency is crucial for organizations dealing with large-scale networks, where the volume of data can be a significant barrier to effective monitoring and threat detection.
The Advantage of Machine Learning: The use of advanced machine learning algorithms in ElastiFlow enables the system to establish baseline patterns of network traffic and subsequently identify deviations from these patterns. These algorithms are trained to recognize subtle signs of insider threats, such as unusual data access patterns, irregular data transfer volumes, or connections to suspicious IP addresses. For example, if an employee suddenly starts accessing large amounts of data at odd hours, machine learning algorithms can flag this activity for further investigation.
A Proactive Approach to Insider Threats
The Tesla data breach is a stark reminder of the importance of internal network visibility in combating insider threats. ElastiFlow, offering both raw unsampled NetFlow and machine learning-based anomaly detection, represents a significant advancement in the fight against these hidden dangers.
Organizations seeking to bolster their defenses against insider threats would do well to explore ElastiFlow. The SEC now requires material cybersecurity incidents to be reported within just four days of detection, including a confirmation of the breach and an impact analysis on financials and operations. Only unsampled NetFlow data provides the deep network explorability required to see which systems were affected by a breach. By adopting an advanced traffic monitoring tool, such as that offered by ElastiFlow, companies can significantly enhance their ability to detect and respond to insider threats, safeguarding their assets, reputation, and stakeholders.
For more information on ElastiFlow and its capabilities in combating insider threats, visit ElastiFlow's website. In the ongoing battle for cybersecurity, staying one step ahead of potential threats, especially those from within, is not just a strategy; it's a necessity.
Stay connected
Sign up to stay connected and receive the latest content and updates from us!